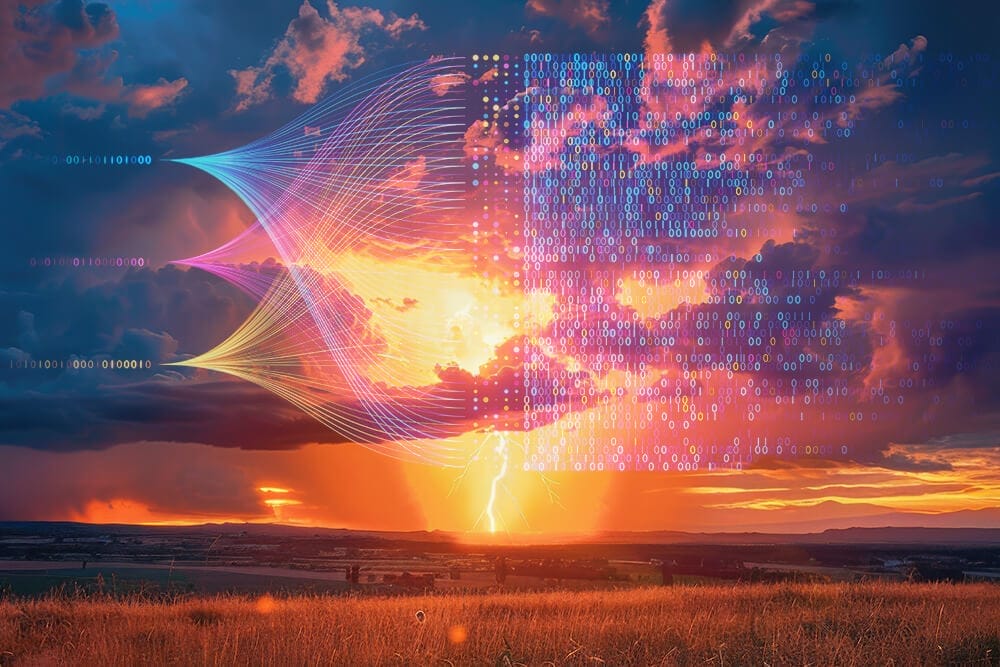
AI in Weather Forecasting
AI in Weather Forecasting: The Future of Weather Prediction
The increasing complexity and unpredictability of global weather patterns necessitate more advanced forecasting methods. As we face new norms of extreme weather, weather forecasting technology must continue to adapt to new weather dynamics and the sheer volume of data generated daily. As a result, there is a pressing need for innovative solutions to enhance the accuracy and efficiency of weather predictions.
Artificial Intelligence (AI) has emerged as a transformative force in meteorology, offering the ability to process vast datasets and generate accurate forecasts with unprecedented speed and precision. By leveraging AI, meteorologists can now harness the power of machine learning algorithms, neural networks, and deep learning models to improve their forecasting capabilities. These technologies not only handle large volumes of data but also uncover patterns and relationships within the data that traditional methods might miss.
This guide aims to delve into the mechanics of AI in weather forecasting, explore its advantages and limitations, and envision the future of AI-driven weather prediction. We will examine the different AI systems used in meteorology, the process of training AI models, and the specific benefits AI brings to weather forecasting. Additionally, we will address the limitations and challenges of AI in this field and discuss how organizations can leverage AI to stay ahead in a world of increasingly volatile weather patterns.
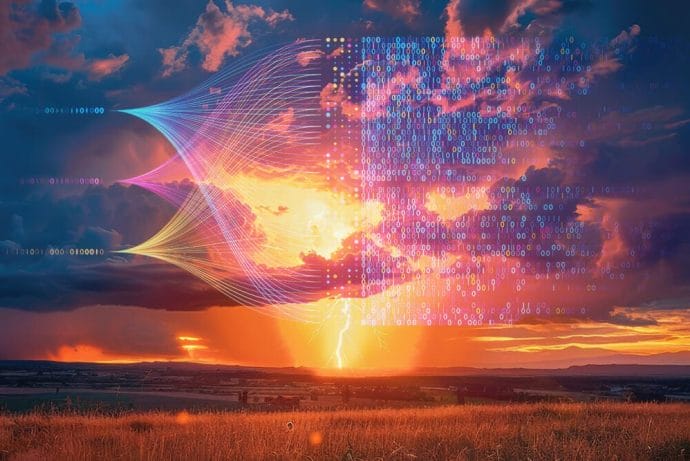
Main Takeaways
- AI significantly enhances weather forecasting accuracy by analyzing large datasets and rapidly identifying patterns.
- Machine learning, neural networks, and deep learning are key AI technologies transforming meteorology.
- AI provides longer lead times for severe weather events, improving preparedness and response.
- Real-time data processing by AI ensures up-to-date weather forecasts for various industries.
- Challenges such as data biases and computational demands need addressing to optimize AI in forecasting.
What is AI?
Artificial Intelligence (AI) refers to the simulation of human intelligence processes by machines, especially computer systems. These processes include learning (acquiring information and rules for using it), reasoning (using rules to reach approximate or definite conclusions), and self-correction. AI encompasses a broad range of technologies that enable machines to perform tasks that typically require human intelligence, such as problem-solving, understanding natural language, recognizing patterns, and learning from experience.
The Different Systems of AI?
AI, in its broadest sense, refers to any machine that can mimic cognitive functions such as learning and problem-solving. It includes a variety of techniques and approaches designed to create intelligent systems capable of performing tasks without explicit human intervention.
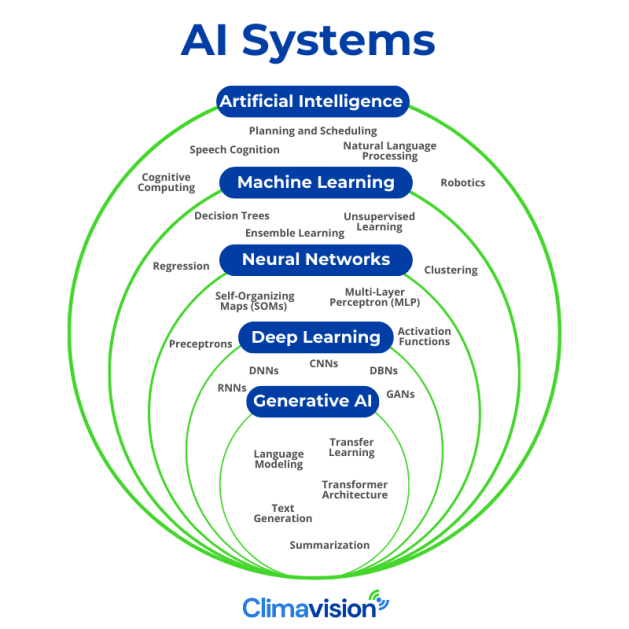
Machine Learning?
Machine Learning (ML) is a subset of AI that involves the use of algorithms and statistical models to enable systems to improve their performance on a specific task with experience (data). Unlike traditional programming, where specific instructions are coded, ML allows systems to learn from data and make decisions based on it.
Neural Networks?
Neural Networks are a series of algorithms that attempt to recognize underlying relationships in a set of data through a process that mimics the way the human brain operates. They consist of interconnected nodes (neurons) that process information in layers.
Deep Learning?
Deep Learning is a subset of ML involving neural networks with many layers (deep networks) that can learn and make intelligent decisions on their own. These models are particularly effective at handling large and complex datasets.
Generative AI?
Generative AI involves algorithms that can generate new content, including synthetic data, by learning from a dataset. These models can create realistic simulations and augment training datasets.
How Does AI Weather Forecasting Work?
AI weather forecasting involves several key steps to transform raw data into accurate predictions:
- Data Collection and Preprocessing: Historical weather data from various sources, such as satellites, weather stations, and sensors, is collected and preprocessed. This data includes temperature, humidity, wind speed, pressure, and other relevant variables.
- Training AI Models: The preprocessed data is used to train AI models. During training, the models learn to identify patterns and relationships within the data. This process involves feeding the data into machine learning algorithms or neural networks, which adjust their parameters to minimize prediction errors.
- Machine Learning and Deep Learning Techniques: These techniques play a crucial role in analyzing complex weather patterns. Machine learning algorithms can identify correlations and trends in the data, while deep learning models can capture intricate, non-linear relationships.
- Integration of Data Sources: AI models integrate data from multiple sources, such as satellite imagery and ground-based sensors, to create a comprehensive view of weather conditions. This integration allows for more accurate and detailed predictions.
- Processing and Analyzing Massive Datasets: AI technologies are designed to handle and process massive datasets efficiently. They can analyze large volumes of data in real-time, providing timely and accurate forecasts.
Breakdown of AI Systems in Weather Forecasting
AI systems used in weather forecasting employ predefined rules to simulate the decision-making ability of human meteorologists. These systems can interpret weather data and generate forecasts by applying rules derived from human expertise. They act as digital assistants, helping meteorologists make more informed decisions by automating routine analysis and focusing on complex interpretations.
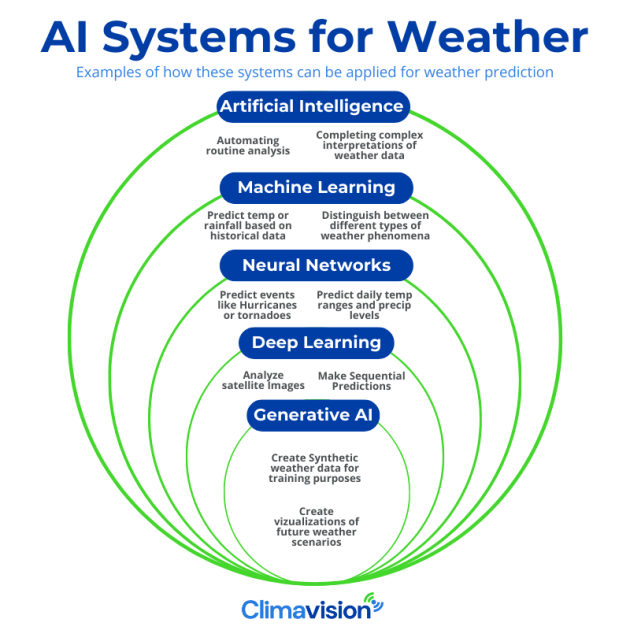
Machine Learning (ML)
Machine learning algorithms analyze historical weather data to identify patterns and make predictions. For instance, regression models can predict temperature or rainfall based on past data. ML can also be used to improve the accuracy of existing weather models by learning from the discrepancies between predicted and observed weather. By continuously updating their knowledge base, these algorithms refine their predictions over time, becoming more reliable and accurate.
Examples:
- Regression Models: Predict temperature or rainfall based on historical data. These models identify trends and relationships between different weather variables.
- Classification Algorithms: Distinguish between different types of weather phenomena, such as clear skies, rain, or thunderstorms, by learning from labeled data.
Neural Networks
Neural networks can model complex, non-linear relationships in weather data. For example, a neural network could be trained on historical weather data to predict the likelihood of severe weather events, such as hurricanes or tornadoes. These networks can capture the intricate dependencies between different weather variables better than traditional statistical methods, enabling more precise and nuanced forecasts.
Examples:
- Predicting Severe Weather Events: Neural networks can model complex, non-linear relationships in weather data to predict events like hurricanes or tornadoes. They learn from historical patterns and improve their predictions over time
- Temperature and Precipitation Forecasting: Neural networks can accurately predict daily temperature ranges and precipitation levels by analyzing past weather data and current atmospheric conditions.
Deep Learning
Deep learning models, such as convolutional neural networks (CNNs), can analyze satellite images to detect and track weather patterns. These models can identify cloud formations, storms, and other meteorological phenomena with high accuracy. Recurrent neural networks (RNNs) can also be used to make sequential predictions, such as forecasting weather over several days based on current conditions and historical data. These deep learning techniques are particularly valuable for understanding and predicting dynamic weather systems.
Examples:
- Convolutional Neural Networks (CNNs): Analyze satellite images to detect and track weather patterns. CNNs can identify cloud formations, storms, and other meteorological phenomena with high accuracy.
- Recurrent Neural Networks (RNNs): Make sequential predictions, such as forecasting weather over several days based on current conditions and historical data. RNNs are effective at capturing temporal dependencies in weather data.
Generative AI
Generative models, such as Generative Adversarial Networks (GANs), can create synthetic weather data for simulations and training purposes. For instance, GANs can generate realistic weather scenarios to augment training datasets for other predictive models, improving their robustness and performance. Generative AI can also be used to create visualizations of potential future weather conditions based on forecast data, aiding in planning and decision-making for various sectors, including agriculture, transportation, and disaster management.
Examples:
- Generative Adversarial Networks (GANs): Create synthetic weather data for simulations and training purposes. GANs can generate realistic weather scenarios to augment training datasets for other predictive models, improving their robustness and performance.
- Visualizations of Future Weather Conditions: Generative AI can create visualizations of potential future weather scenarios based on forecast data, aiding in planning and decision-making.
AI Weather Models vs. Traditional NWP Forecasting Models
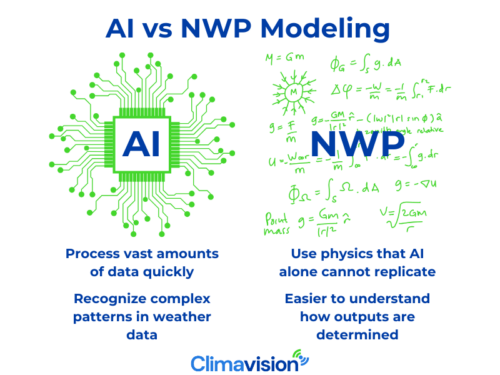
AI Advantages
AI models offer advantages that traditional weather forecasting methods may lack:
- Data Processing Speed: AI can process vast amounts of data quickly, providing faster and more accurate forecasts. Traditional methods often involve manual data analysis and can be time-consuming.
- Pattern Recognition: AI excels in recognizing complex patterns in weather data. This capability allows AI to make more accurate predictions by understanding the nuanced interactions between different weather variables.
AI Limitations
While AI offers numerous benefits, there are challenges and limitations in its application to weather forecasting:
- Data Biases: AI models can inherit biases present in the training data, leading to skewed predictions. Ensuring diverse and representative datasets can help mitigate this issue.
- Computational Resources: AI models require significant computational power and resources for training and deployment. Investing in robust infrastructure and optimizing models for efficiency can address this challenge.
- Understanding Physics: AI alone cannot fully understand the physics of weather systems, while NWP models can. AI models rely on data-driven approaches and may overlook fundamental physical principles governing atmospheric processes and therefore must be trained on NWP models.
- Black Box Problem: The decision-making process of AI models can be opaque, making it difficult to understand how outputs are determined. This lack of transparency can be a barrier to trust and acceptance among meteorologists and decision-makers.
The Advantages of Incorporating AI with NWP Forecasting
While AI and NWP models may have their shortcomings alone, together they significantly enhance weather forecasting in several ways. For example, as Climavision’s Chief NWP Scientist Peter Childs explained to Middle Tech Podcast’s Logan Jones, while AI may not be able to understand physics alone, it can help advance NWP modeling by uncovering better parameterizations.
Climavision uses cutting edge AI technology to its fullest extent to create AI models like our Horizon AI Subseasonal to Seasonal model as well as bolstering the speed and accuracy of our NWP models Horizon AI Global, Pointand HIRES. With AI and NWP expertise combined, Climavision can offer the entire timescale horizon of forecasting from real time radar coverage to long term forecasts as much as two years out. From data assimilation and quality control to generating hundreds of ensemble forecasts, we have incorporated AI into every step of the forecasting process so that we can deliver forecasts tailored to specific use cases with greater speed and verified accuracy.
Enhanced Forecast Accuracy
AI algorithms analyze vast datasets from satellites, weather stations, and other sensors to identify patterns and trends. By refining predictive models, AI leads to more accurate weather forecasts. For example, AI can improve the accuracy of temperature, precipitation, and wind predictions by learning from NWP models and continuously updating based on new information.
Factors influencing the accuracy of AI forecasts include the quality of data, the sophistication of the AI model and its training, and the specific weather phenomena being predicted. High-quality, diverse datasets and advanced models are crucial for achieving accurate predictions. Moreover, AI’s ability to learn and adapt from past performance helps improve forecast accuracy over time.
Climavision is pushing the envelope of accuracy across our entire Horizon AI Forecasting suite. For example, our Horizon AI S2S model is 30% more accurate globally and 100% more accurate for specific locations versus the ECMWF. The improved accuracy is due to our S2S model being fueled by decades of back testing data, training on millions of observations plus proprietary climate simulations, and being informed on 500 different ensembles. Additionally, Climavision ensures the Horizon AI S2S model avoids the black box problem by producing forecasts that are explainable based on easily interpretable neural networks developed entirely in-house.
But don’t take out word for it, see for yourself. We have built a fast, easy to use API for our Horizon AI S2S model that you can access to see accuracy metrics (CRPSS, ACC and MAE) for past forecasts as well as a back tested forecast (i.e., what our forecast would have been if you queried it on this day) and the observed weather, for a particular latitude and longitude pair, or for a global forecast. Read the API documentation here.
Data Analysis and Integration for NWP models
AI algorithms not only help with AI weather models, but they also benefit NWP models as well. AI systems excel at processing and analyzing large volumes of data from various sources, such as weather satellites, weather stations, and ocean buoys. AI algorithms can integrate and interpret this vast amount of data, identifying patterns and correlations that humans might overlook. By integrating different data sources, AI improves the overall accuracy of weather models.
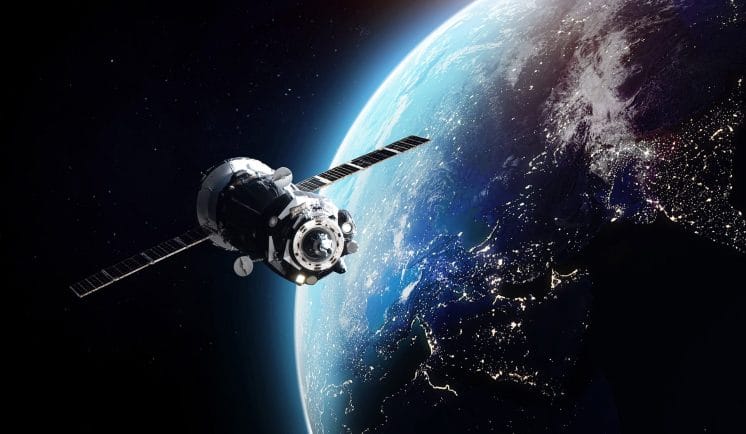
At Climavision, we are leveraging AI algorithms to process and analyze vast amounts of data to integrate more than 1.5 billion global observational datasets daily into our NWP models. These datasets come from novel sources such as space-based datasets. The ability to utilize these novel, massive datasets give our partners more insight into what is happening, even in remote areas in real time. In fact, through our meticulous data processing and rigorous quality control measures, we elevate the usable observational data to over 20%, a stark contrast to the mere 3% provided by government sources. The more data there is to input into weather models, the more accurate the model outputs will be.
Not Just Any AI Will Do
Artificial Intelligence has revolutionized the accuracy and efficiency of weather data and forecasting, benefiting numerous industries. Accurate predictions enable early warnings and preparedness for severe weather events, protecting lives and property. But not all AI weather forecasting is made equal. It’s important to consider the following:
- Is the AI-generated weather forecast available now? While some companies have recently announced new AI capabilities, they don’t have any forecasts available for the market.
- If they are available, how much more accurate are the AI forecasts than government model forecasts?
- How are the AI forecasts accuracy verified and how often?
- Are the forecasts probabilistic so that you can see the entire range of possibilities with their associated likelihoods?
- Are the forecasted results of the AI model explainable so that you can easily determine how the forecast reached those conclusions?
Future-Proof Your Decisions with Climavision
In a world of increasingly volatile and unpredictable weather patterns, AI in weather forecasting is invaluable. Climavision stands at the forefront of this technological revolution, utilizing AI capabilities in data processing and modeling. Adopting Climavision’s solutions empowers businesses, governments, and communities to make informed, proactive decisions in the face of weather uncertainties.
Climavision’s advanced weather forecasting solutions combine cutting-edge technology with extensive meteorological expertise. By leveraging AI, Climavision provides accurate, timely, and reliable weather forecasts that help organizations navigate the challenges and opportunities posed by climate change and extreme weather events.
Climavision’s AI-powered weather forecasting solutions enable proactive decision-making, allowing businesses and communities to prepare for and respond to weather events more effectively. Whether it’s optimizing agricultural practices, ensuring safe transportation, or managing risks in energy production or commodity trading, Climavision’s innovative approach to weather forecasting provides the tools needed to stay ahead in an unpredictable world.